Unleashing the Power of Data Annotation: Essential Tools and Platforms
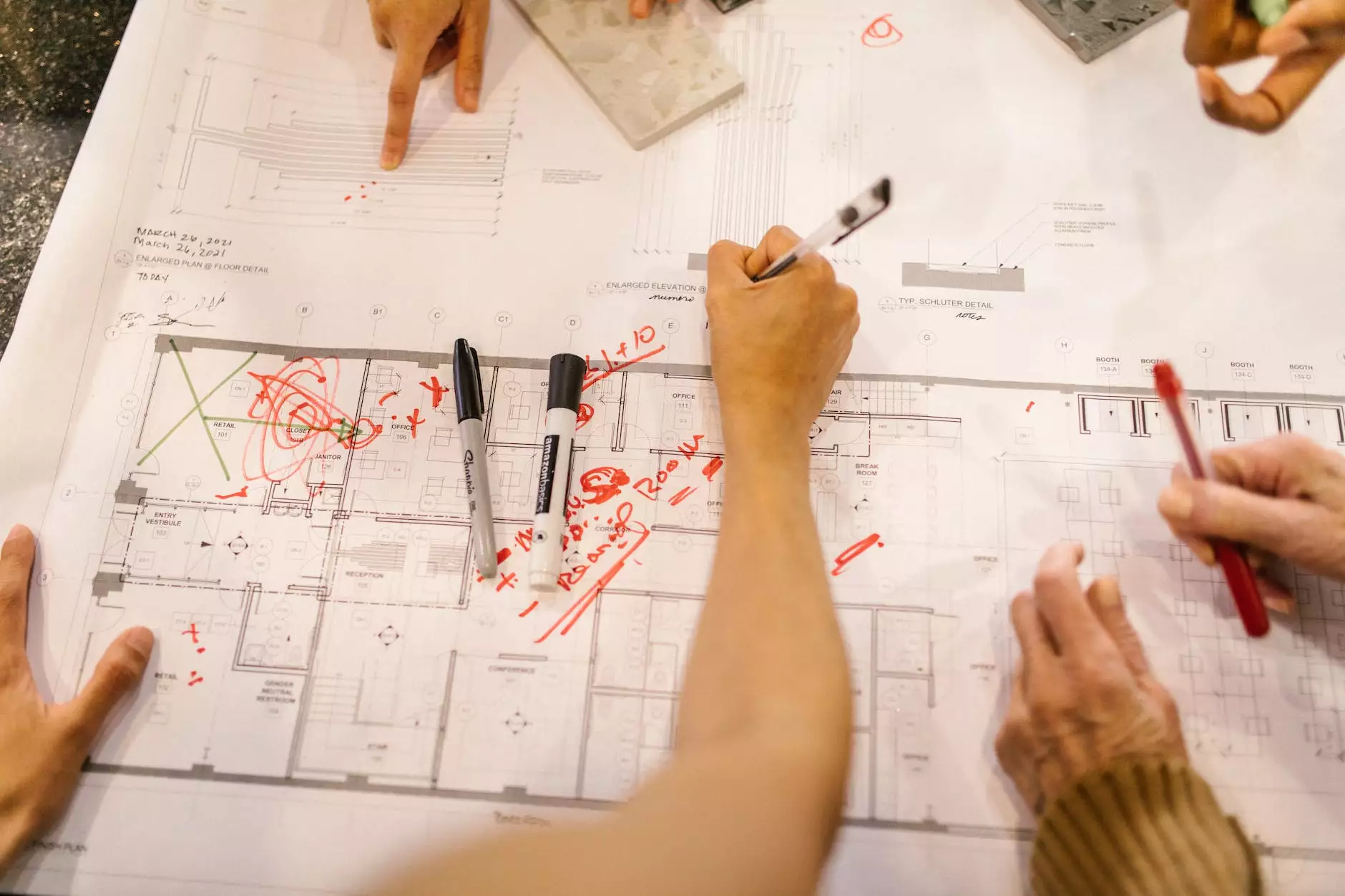
In today's data-driven world, businesses are constantly seeking innovative ways to harness the vast amounts of information available to them. The rise of artificial intelligence (AI) and machine learning (ML) has revolutionized how organizations operate, but these technologies require high-quality data to function effectively. This is where data annotation tools and data annotation platforms come into play.
What is Data Annotation?
Data annotation is the process of labeling or tagging data to provide context, making it understandable for machine learning algorithms. This can include text, images, audio, and video files. The annotated data is then used to train machine learning models, enabling them to make predictions or perform tasks with accuracy.
Types of Data Annotation
- Image Annotation: This involves labeling images with relevant tags or categories, such as bounding boxes around objects in photos. It's crucial for computer vision applications.
- Text Annotation: This includes tasks like sentiment analysis, entity recognition, and part-of-speech tagging in natural language processing (NLP).
- Audio Annotation: Annotating audio clips involves labeling speech, sounds, or identifying specific audio signals, which is essential for speech recognition systems.
- Video Annotation: This entails labeling video content frame-by-frame or segmenting videos for object detection and tracking.
The Importance of Data Annotation for Businesses
In the realm of artificial intelligence, the phrase "garbage in, garbage out" holds true; the quality of the output is directly dependent on the quality of the input data. Proper annotation of data is essential for several reasons:
- Improves Model Accuracy: Well-annotated data ensures that machine learning models learn the right features, enhancing their predictive power.
- Reduces Bias: Comprehensive labeling helps in identifying and mitigating biases in AI models, leading to fairer outcomes.
- Enables Versatility: A variety of annotated datasets can allow models to perform multiple tasks, increasing the scope of applications.
- Facilitates Compliance: Proper data annotation ensures that businesses remain compliant with regulations regarding data usage and privacy.
Choosing the Right Data Annotation Tool
Selecting an appropriate data annotation tool is critical for businesses aiming to enhance their AI and machine learning capabilities. Here are some essential factors to consider:
Key Features of Data Annotation Tools
- User-Friendly Interface: A simple and intuitive interface makes it easier for teams to annotate data efficiently.
- Scalability: As businesses grow, their data needs change. Choose tools that can scale to suit increasing data volumes.
- Integration Capabilities: The tool should seamlessly integrate with existing systems and workflows for efficient data management.
- Collaboration Tools: Look for platforms that allow team collaboration, as data annotation often requires multiple inputs.
- Quality Control Features: Implement features that allow for validation and review processes to ensure data quality.
Top Data Annotation Platforms in the Market
Several data annotation platforms have gained popularity for their robust features and capabilities. Here are some of the frontrunners:
1. Keylabs.ai
Keylabs.ai stands out as a premier data annotation platform offering a suite of tools for efficient data labeling. Its machine learning-assisted annotation processes expedite the labeling workflow while maintaining high accuracy. The platform supports various data types, making it versatile for diverse business needs.
2. Amazon SageMaker Ground Truth
This platform provides tools for building highly accurate training datasets efficiently. SageMaker Ground Truth integrates with Amazon’s other services, enhancing the power of its annotation tools.
3. Labelbox
Labelbox offers a collaborative interface that enables teams to work on data annotation projects together in real-time. It supports various data types and has built-in tools for quality control.
4. Supervisely
Supervisely focuses on providing tools specifically for computer vision tasks, enabling easy annotation of images and videos. It offers a rich ecosystem of machine learning tools.
5. Scale AI
Scale AI is known for its high-quality annotated datasets. It uses a combination of human annotators and machine learning models to deliver effective results quickly.
Best Practices for Effective Data Annotation
To achieve optimal results from data annotation, businesses should adopt certain best practices:
1. Define Clear Annotation Guidelines
Establishing clear instructions and guidelines is crucial for consistency in data labeling. Well-defined criteria help annotators understand what is required, resulting in improved accuracy.
2. Implement Training Sessions
Conduct training sessions for annotators to familiarize them with the tools and the specific requirements of the project. This enhances their efficiency and effectiveness.
3. Utilize Quality Assurance Mechanisms
Incorporate review processes and quality checks to ensure the annotated data meets the necessary standards. Regular audits can help identify and mitigate potential errors.
4. Encourage Team Collaboration
Utilize collaborative platforms to promote communication among team members. Encouraging discussions and feedback can lead to better data annotation quality.
5. Regularly Update Practices
Data annotation practices should evolve over time. Regularly review and update guidelines based on feedback and technological advancements to maintain efficiency.
Conclusion
As the demand for machine learning and AI solutions continues to rise, the importance of high-quality data cannot be overstated. Data annotation tools and data annotation platforms like Keylabs.ai play a pivotal role in ensuring that businesses can leverage their data effectively. By following best practices and choosing the right tools, organizations can significantly enhance their AI capabilities, paving the way for innovation and success in the modern business landscape.
Investing in proper data annotation is not just an option—it’s a necessity. It empowers businesses to transform raw data into actionable insights, driving growth and maintaining a competitive edge.
https://keylabs.ai/